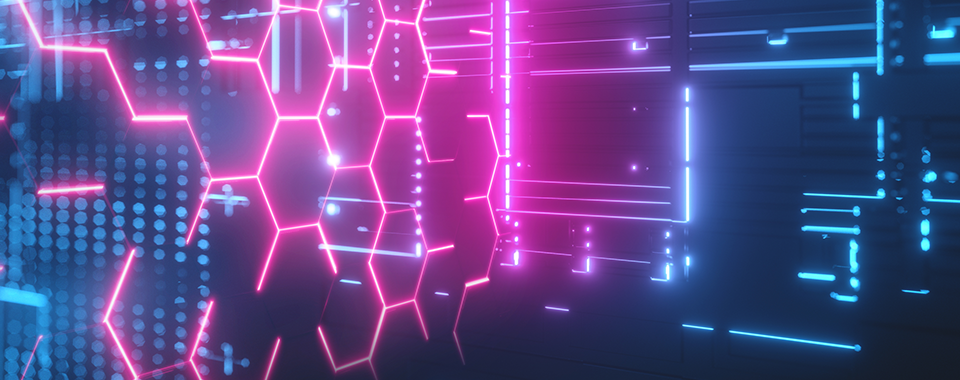
When you buy AI, you are not buying a software license!
In U-Hopper we have spent the last few years building and selling custom Artificial Intelligence solutions (mostly classifiers and regressors, or variants thereof) to our customers, which include manufacturing companies, retail banks, transportation operators, pharmaceutical and chemical industries, and some other ones. During this journey, we have learned (often the hard way!) some best practises on how to market AI solutions and how to ensure a smooth communication with our customers. Yet, from time to time things do not work as expected, and we run into issues. In particular, we have found two common misunderstandings that may happen – and that prevent a successful project completion. Here is our experience – and a few tips on how to handle the situation.
No data, no party
The first issue relates to data availability. Some customers do not understand that, unless we get good data (good data = a LOT of clean data), our AI will not perform well. It has happened to us a few times to get blamed “Your solution does not perform as expected!” (well, in some cases the customer used a somewhat less sweet wording…). Not nice, of course – but it turned out that the problem in all such cases was that the customer did not provide us good data to train our methods. And no matter how good our (or: your) data scientists are, they do not make miracles. And yes, training a good AI with poor, few data is actually a miracle (so it is unlikely to happen, and if it happens you should not believe it!).
How to avoid it? Good communication is key here. We discovered that the best way is to organise at the very beginning a corporate workshop on AI with the key customer’s staff members involved in the project. In this way we could build a common understanding of how AI works – and ensure people at the customer’s side understood clearly the importance of data and the fact that they have to provide data, that is not on us! We also learned that it is good practice to cater explicitly in the contract for data availability – just in case things turn out really bad, at least you have some backup.
Did someone say software license?!
The second issue relates to retraining. When you buy an AI, you actually buy a form of living digital organism. And, as with anything alive, you need to take care of it. In our case (which is 99% Machine Learning-based solutions), this means that, from time to time, you will have to re-train the AI model, i.e. you will need to ‘feed’ it with new data and let it – roughly speaking – reconfigure itself. After that, you will have to deploy the new version of the model and then you will be good to go.
How often do you need to do this process? It depends on how much data is changing (if, let’s say, your data is about sales you may have some seasonality effect, etc.). But let’s say, as a rule of thumb, that every 6 to 12 months you will have to retrain your model. Does this mean that you have to re-do everything from scratch? Of course not. 90% of the work will already be done – you will just have to let the model recalibrate its internal parameters.
However, it is important to understand that with AI you are never truly done. Our mantra with customers is “This is not like buying a software license“: it is not a one-off action, that once done you can forget about. Again, here communication is key, in order to make sure expectations from the customer are actually aligned with what we (or: you) deliver. We typically offer one or two re-trainings as a free perk in the package – this ensures that they are carried out and allows us to build a longer-term relationship with the customer.
Root cause analysis
You might have noticed that both issues can be traced back to two common – and interwoven – causes. First, a poor understanding of how AI actually works. Second: the lack of a data culture in the company. Let us discuss them shortly.
The first one – poor understanding of AI – is actually easy to understand. Our customers are not AI experts. They build things (from nuts & bolts all the way to complex chemical plants) or deliver services (financial, information, etc.). What they know about AI is what they get from mainstream media. And so many end up believing that AI is actually a sort of ‘black magic’ that can solve any problem. But AI is not magic! It employs maths and statistics to learn from facts – i.e. from DATA!
Which takes us to the second cause of the problem: the lack of data culture. Still too many companies fail to accept to look at data to understand – truly! – how they are doing and what is not working, and to use that data to make decisions, in an effort to improve business KPIs. This ‘fear of data‘ is something quite common in mid-level management, where people are often afraid that AI will diminish the value of their experience (and to their perceived value to the company). No silver bullet here, but the inclusion of corporate workshops and (ideally) trainings as part of the offer is something that definitely helps in smoothing such issues.
Last, but not least, another factor to be considered is the prevailing mindset in the IT department of many (non-IT) companies. In most cases, our customers are indeed productive industries or service-based companies. In both cases, their internal IT department is mainly concerned with business continuity, by ensuring things do not break. So they are naturally risk-averse, and may create barriers to innovation. Furthermore, they typically do not develop solutions internally, but they mostly buy from external providers… hence the equation software = license. While getting our customer’s IT aligned with our AI approach is a lengthy and often cumbersome process, it is a necessary step if you want to build a long-term, sustainable, business relationship.
Did you enjoy this article?
Enter your e-mail address to receive our newsletter!
PS: don’t worry, we hate spam just as much as you do. We’ll send you just a couple of emails a year with a collection of the most interesting articles, it’s a promise!